These are the most important AI trends, according to top AI experts
Somewhat in the shadow of the (often) overhyped metaverse and Web3 paradigms, AI seems to be developing at great speed. That’s why we asked a group of top AI experts in our network to describe what they think are the most important trends, evolutions and areas of interest of the moment in that domain.
.png)
All of them have different backgrounds and areas of expertise, but some patterns still emerged in their stories, several of them mentioning ethics, the impact on the climate (both positively and negatively), the danger of overhyping, the need for transparency and explainability, interdisciplinary collaborations, robots and the many challenges that still need to be overcome.
But let’s see what they have to say, shall we?
One more thing, if you’re interested in what artificial intelligence could mean for your business, maybe you’d like to know that we are organizing The (next) era of AI Tour in London in May 2023, which will be moderated by Geertrui Mieke De Ketelaere (one of the writers below).
It’s time to move beyond the hype
By Bernie Caessens, Lead scientist at Poolstok

Technology is ubiquitous in all parts of everyday life. If one follows the popular media, technological advances take on epical and mythological proportions. There is nothing wrong with healthy enthusiasm. But false claims about technology really can be harmful in many ways. We really should stop going for the headline and take time to think things through, especially when it comes to technology. Especially when we are hoping to solve the real problems like climate change and disease and famine through technology.
Take AI for instance. Despite the many successes of deep neural networks in the past decade, and the obvious applications and gains we tend to forget that this is not AI, by far. Neural networks have not (yet) learned to recognize faces, write poetry or play games. They optimize the association of pictures to labels, the prediction of a word in a sentence or the association of pixel positions to joystick positions. There is no reasoning or thinking involved. We are no closer to thinking machines than we were in the previous century, and yet, we act as if we do.
One consequence is the rapid commoditization of this technology. In nearly every business area some vendor is offering solutions ‘based on AI’ or with ‘AI included’. Unfortunately in many cases, artificial intelligence included means true intelligence excluded. At its best this causes just a waste of resources, at its worst it leads to bad decisions causing damage, like discrimination when hiring. The potential harm is serious. For people, business and society. This is not new, this is not unknown. There are and have been serious critics on the current approach to AI (e.g. https://garymarcus.substack.com/), and there is serious science around this. Let’s take the time to discuss it, to put things in their proper perspective.
In fact, in the previous paragraphs, you could probably substitute the word AI for any other technology on the rise. The text would still make sense. The time has come to treat technology more carefully and thoughtfully. Decoupled from the rush of the economic machine. As humans, not as individuals, we are in for the long haul. There are no shortcuts in the long run. Let’s not place our hope in hypes.
Dream big
By Ann Dooms, Professor Math & Data Science at VUBrussel

The most popular artificial intelligence technique must be the so-called neural network that loosely models the behavior of the neurons in our brain with mathematical transformations. Although invented around 1950, neural networks are currently booming because of the ever increasing computing capacity and the massive amount of digital data allowing them to learn by example.
We can teach a computer to see by showing it lots of labeled photos. As a digital image is actually a large table filled with numbers describing the color of the pixels, the neural network can perform calculations on these in order to recognize shapes on the picture. This boils down to learning suitable weighted combinations of the input numbers by solving a very large nonlinear system with hundreds of thousands of parameters. Once the network is trained, it can recognize those shapes on new unseen photos. Hence by showing it lots of examples of cats, dogs, cars etc, it will learn the characteristic features of each class it has seen. The network “looks” in a granular way: by identifying lines and their compositions it is able to distinguish texture from content. When applied to, for example, scans of paintings, such a network can quickly grasp the style of an artist and even transfer it to other pictures while keeping their content untouched.
We can also teach a computer to understand a language by showing it lots of digital texts. By making connections between words and the surrounding text, the neural network can learn their meaning and even context. The result is language model that can generate texts, from essays to poems. The real magic happens when we combine such neural networks with those that can see resulting in an algorithm that can generate images from a textual description. What started with artificially generated armchairs in the shape of an avocado, was quickly followed by fascinating synthetic products such as sneakers with patterns from famous artists like Frida Kahlo or Keith Haring and fake paintings, like that of a seal with a pearl earring in the style of Vermeer.
By now we have several big players in the field, e.g. DALL-E, Stable Diffusion or Midjourney, allowing you to turn your wildest phantasies into images in the cloud or on your own (powerful) machine. Recently, Meta jumped the wagon too and released its Make-a-video from a text prompt. These impressive content creators shed a whole new light on the creative industry, but also open the door to misuse. Seeing is no longer believing, but let that not keep you from letting your imagination run wild.
Homo Roboticus
By Bram Vanderborght, Brubotics, Vrije Unveirsiteit Brussel and imec

Europe starts from a strong position in robotics. In industrial robotics, it controls around one third of the world market, while in the smaller professional service robot market European manufacturers produce 63% of the non-military robots. Many robots do not use artificial intelligence algorithms for the control of their behaviour. Their control is dependent on known models, and, based on these models, calculations and input data, a complex algorithm defines the output and hence the behaviour of the robot. For example the impressive robots of Boston Dynamics do not use machine learning.
However, as such the system cannot learn. Artificial intelligence systems provide robotics application new impressive perspectives, but also come with complex challenges. For aspects like vision and speech recognition, deep learning has drastically outperformed traditional methods. These approaches typically require massive amounts of labelled data and computational power. Although data is typically abundant in robotics, labelling is sparse and expensive. For the control of robots, reinforcement learning often requires significantly more iterations than are feasible on real systems.
Therefore, a lot of the learning work is done in virtual environments, with challenges to transfer to the real world. Moreover, robots are interacting with the real world and challenges exist how to learn in a safe way, for the humans, for the environment and for the robot itself. Also explainability is important, so humans are able to understand and hence trust how the AI system came to its decisions.
Both methods, model-based control and learning methods, have disadvantages and advantages, and combining the best of both worlds is probably an interesting solution. Moreover like in animals and humans, due to millions years of evolution, the bodies are optimized for the tasks and the environment. Through a smart design with the use of new materials, actuators and sensor, part of the computational intelligence can be outsourced to the embodied intelligence of the robot body.
According to the paradox of Moravec “AI can quickly learn to perform tasks considered "difficult" by humans”, such as complex statistics and analysis. However, on the other hand, tasks that are trivial for humans, are often still exceedingly difficult for computers and robots.” Therefore instead of replacing humans by robots, there has been a lot of focus on developing collaborative robots to exploit these complementary strengths. History teaches us that technology has the power to strengthen economic growth and transform societies. People and robots will have to live together, and this raises technical, social and ethical questions. How do we want to live as humans in the future? In our Book “Homo Roboticus” we want to bring these aspects on the table and propose a draft for an inclusive robot agenda.
We need more interdisciplinary research

We live in exciting times! We have seen vast progress in artificial intelligence (AI) in recent years. Especially when it comes to narrow AI - an AI algorithm designed to perform a specific task - these systems often outperform humans. An excellent example is EfficientZero, an AI algorithm that reaches superhuman performance on Atari games after as little as 2 hours of gameplay. Also, we are well acquainted with AI translators and copy-editing applications. Furthermore, it is handy that we can search for faces, cities or objects in our digital photo library. We have even heard about doctors relying on AI-based tools that assist them with clinical diagnostics. Likewise, researchers design AI algorithms to control plasmas in tokamak nuclear fusion reactors. On the fun side, some of us have experimented with generating pictures (that we call “art”, wink) with Dall-e 2 or Stable Diffusion. However, the long-awaited robot butlers and fully self-driving cars are not yet there due to the high variability in our physical world.
As an AI and robotics researcher, the application of AI algorithms to physical applications (such as robots) intrigues me. To advance, I see the necessity of interdisciplinary research. Brain research -- which is the topic of the Human Brain Project -- on cellular resolution might lead to new methodologies to design and train neural networks that might help us move us beyond narrow AI. Furthermore, this can also lead to new, more energy-efficient (neuromorphic) chips so that robots can operate longer without draining their battery.
In my lab, we work hard to build and train a new generation of collaborative robot butlers that can help people with everyday tasks. I am convinced that the AI capabilities in these robots are intertwined with their sensorimotor capabilities. Consequently, our lab focuses on robot morphology, control, perception and human interaction. To give a specific example: I collaborate with Dominique Adriaens, an evolutionary biologist, to design and control a new robotic manipulator based on the seahorse tail. Therefore we started an evolutionary design process inspired by the morphology of a seahorse. In this process, the manipulator is gradually shaped to give it more and more functionality. At the same time, the controllers for these bio-inspired robotic manipulators are trained, i.e., so-called co-learning of embodiment and control. We are convinced that this will lead to a versatile robotic manipulator that is both strong and flexible, which might be helpful in the next generation of robot butlers.
New AI technologies enable exciting applications. In the examples above, we focused on technological aspects. However, AI's progress also poses new social, economic and ethical puzzles. Answers to these questions will not come from those who design these systems, hence this plea for truly interdisciplinary research. To enable this, I'm convinced that everyone should be literate - by which is meant a basic understanding and not full expertise - in STEM (Science Technology, Engineering and Mathematics), including Computer Science. Education plays a vital role in this. For this reason, I founded Dwengo vzw. At Dwengo, we develop innovative educational resources and provide them to teachers. In all our projects, we explain basic concepts of AI and STEM starting from a societal challenge. In that way, we want to inspire not only the next generation of technology creators, but also enable critical thinking about what is to come.
We need to take active steps towards an ethical AI
By Geertrui Mieke De Ketelaere, Adj. Professor Vlerick & Strategic Advisor AI imec (IDLab)
.jpeg)
On October 28, 2022, Belgian Federal Deputy Prime Ministers Pierre-Yves Dermagne and Petra De Sutter as well as Belgian Federal State Secretary for Digitalization Mathieu Michel launched the national convergence plan for the development of artificial intelligence. I think it’s absolutely fantastic that ethical AI takes up a central position in this new plan.
Today, however, too many AI developers and companies still see business and organizational “ethics” as something subjective, or even as a wishy-washy term. But nothing could be less true. Ethical beliefs may differ contextually from person to person, but ethics itself is based on human rights. And these are anything but unclear.
So, can't we just wait for a legal framework, then, and for now just quickly move forward with our innovative developments? I'm afraid not.
Today, there is still too much uncertainty and not enough transparency in the field of data use, AI and autonomous decisions. Even more so, because of the hidden nature of the technology and its rapid developments, the knowledge gap between developer and user only seems to be increasing.
Can't we just follow the well-known practices, such as setting up a "code of conduct" or an "ethical board", as the larger AI players seem to be doing? Unfortunately not. These “ethics-washing” techniques lack any real impact whatsoever.
To help create a society in which our AI systems can gain further trust and thus become more socially accepted by the end user, every company should take these crucial active steps:
- An ethical dialogue
- An ethical framework
- A focus on content
- A focus on the organization
An ethical dialogue
In the design phase, and focusing on their external relationships, companies should initiate a bi-directional dialogue with citizens, in a crystal clear and understandable language. First and foremost, it is essential that everyone in the business, as well as its users, understands how the AI will work in the solution and what its possibilities and limitations will be. Based on this dialogue, it is important that companies find a transparent balance between innovation and ethics before they embark upon the hunt for profit. When they enter the development phase, it is crucial that a company already thinks about the content of the information leaflet that will accompany its AI service or product. You could compare that to the Patient Information Leaflet (PIL) that each medicine package contains, which is actually not a legal requirement, but strongly recommended from a moral perspective and brand experience.
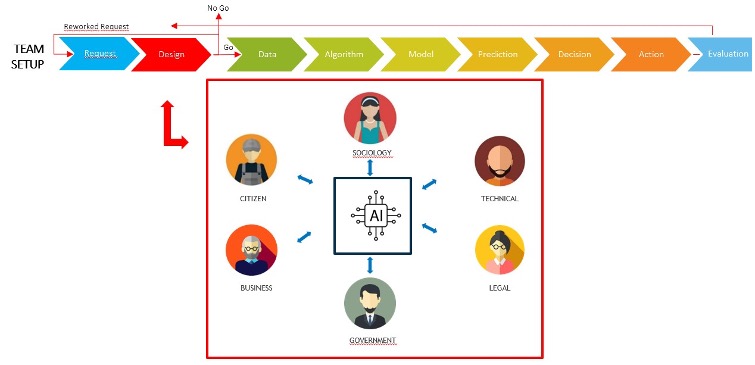
An ethical framework
Once the development project starts, it is important - from an internal focus - that companies can explain how they have developed their systems responsibly in each step of the process. And that they can justify these actions in an understandable language. An ethical framework could be really useful here: one in which a clear distinction is made between the steps required to ethically substantiate the content of the system and the organization of the company to support the ethical development.
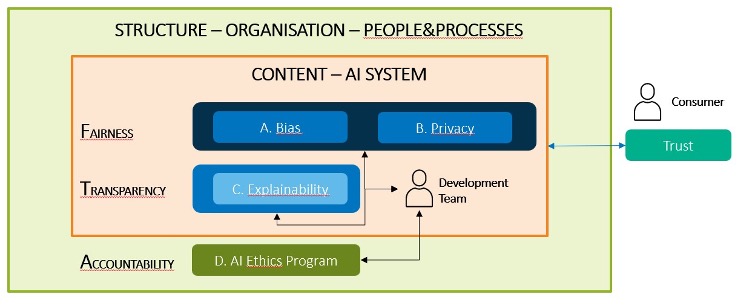
A focus on content
For this purpose, various tools and technologies have already been developed in previous years to ensure points such as fairness and transparency at every stage of the process. Today, we notice that companies that incorporate ethics often limit themselves to the content side, thereby indirectly placing full ethical responsibility with the developers. However, despite some steps in the right direction, this is a bottom-up approach that is not only incomplete but also incorrect in many aspects.
A focus on the organization
Perhaps the most important aspect of ethical AI is that it ought to be the responsibility of everyone in the company. It truly must be ingrained top-down into the DNA of the company. There needs to be an ethical framework that offers crystal clear guidelines on how the entire structure of the organization must operate in an ethically responsible manner. These recommendations must also include clear descriptions of the various responsibilities. Such a vision should be initiated and dispersed from the top down.
These steps, frameworks and processes should sound like music to the ears of any company working with AI and putting ethics high on the agenda.
The role of AI in climate tech
By Jessica Groopman, Director Digital Strategy & Innovation at Intentional Futures
Artificial intelligence is emerging as an unexpected category in climate tech, and a catalyst for mitigation and adaptation.

AI has often been likened to “fire” or “electricity” to illustrate the scope of its potential impacts– opportunities and risks alike. But this analogy resonates even more as it becomes a critical tool for addressing the enormous challenges (and novel solutions needed) for humanity to thrive on a warming planet.
To date, “climate tech” has often referred to categories in renewables, like solar and wind, or connected infrastructure across energy, buildings, and transportation. But increasingly it’s all tech on deck, and AI offers a wide (and growing) range of techniques, software, and hardware with perception, analysis, and predictive capabilities for both mitigation and adaptation.
- To predict and optimize energy loads based on demand, not only in energy grids, but in data centers, cloud workloads, virtual environments, electrified fleets, and through digital twins of smart home and city applications.
- To monitor and mitigate risks to agricultural land and yields, by pulling in a wide range of weather, soil, pests and other datasets to analyze, verify, and improve output while maintaining stable growing conditions.
- To develop new bio-based formulas to displace petrochemical-based materials used in numerous industries. By mining massive genomic and molecular data sets and recognizing patterns, machine and deep learning help “recommend” alternative recipes for compounds and materials that do not require fossil fuel extraction.
- To analyze alternative material footprints to help designers and product teams across industries meet specific product and performance niches, while avoiding over extraction of ecosystems.
- To preserve biodiversity and advance conservation efforts through improved intelligence and accountability. Whether via computer vision-enabled drones or satellite imagery, these proverbial ‘eyes in the sky’ are helping on-the-ground human stewards gain longitudinal data and insights into animal behavior, landscape impacts, risks of poachers, and other human influences.
- To automate circularity and product reuse, machine learning is helping companies across the entire circular value chain. For example, by analyzing excess inventory to resell to the highest value channel based on need, software platforms are helping companies seamlessly triage customer returns, reduce waste, and win revenues.
- To reduce waste and improve recycling, computer vision-enabled robots sorting garbage, or connected waste bins to weight, measure, and analyze materials for more efficient triage, inventory, and waste management prediction.
- To support behavioral changes via personalized nudges, transparency, visualization, and contextual content to build awareness around more conscious consumer decisions, whether deciding what to eat, what to buy, where to recycle, or optimizing which route to take.
These are just a few of hundreds of examples of AI used to address the many challenges facing businesses, communities, and policy-makers to develop a more resilient economy in concert with the planet. This trend is only poised for growth, considering the widespread movement of tech talent into climate jobs, intersection with other AI trends such as cybersecurity or predictive maintenance, the increasing investment in ESG (environmental, social and corporate governance) programs, and the continued rise in both collection and varieties of climate-related data, and their implications for what we measure.
AI for climate: towards environmentally responsible research and innovation
By Mark Coeckelbergh, Professor at Department of Philosophy, University of Vienna
.jpeg)
In contrast to the technologies of the industrial revolutions, which caused unprecedent levels of air and water pollution and darkened the sky of many cities, digital technologies seem clean and environmentally friendly. But during the last years we have started to realize that these technologies are less innocent than they seem.
Consider climate change and artificial intelligence (AI). AI provides a lot of opportunities. Data analysis by AI can help scientists study climate change and AI can create smarter and more economic ways of dealing with energy – something very important in the current energy crisis. But there are also problems. Data centers use energy, and it is known for example that large language models use a lot of energy.
Moreover, even if the software were clean, software is always connected with hardware and infrastructure. And that hardware and infrastructure needs to be produced and maintained, requiring natural resources and thereby impacting the environment. As Kate Crawford has shown in her book The Atlas of AI, for example, the political ecology of AI is dirtier than we think.
If we want to work towards a more sustainable future for people and planet, therefore, we not only need to use the available digital technologies to help us to mitigate climate change and reduce energy consumption; we also need to change the technologies themselves in a more sustainable and climate-friendly direction. We need clever ways to develop and use what I have called AI for climate.
Top-down regulation and government incentives is one way to achieve this; AI policy needs to include an environmental and climate component. But we cannot and should not wait until politicians take action. Environmentally responsible research and innovation is something developers, universities, and companies can do already now. People should be stimulated to use their creativity to make better technology, with “better” meaning: better for people and better for climate and environment.
Next to integrating environment and climate concerns in our thinking about the ethics and politics of AI, which I have tried to do in my work on AI ethics and the political philosophy of AI, we need concrete proposals that can be integrated in development and management practice. An example is “sustainability budgets’: a practical solution that gamifies the problem, respects the autonomy of developers, and can be integrated in software design and governance of AI.
AI can have a bright future if we make sure it’s a green one.
We need to broaden the conversation about climate change
By Lode Lauwaert, Professor of Philosophy of Technology at KU Leuven
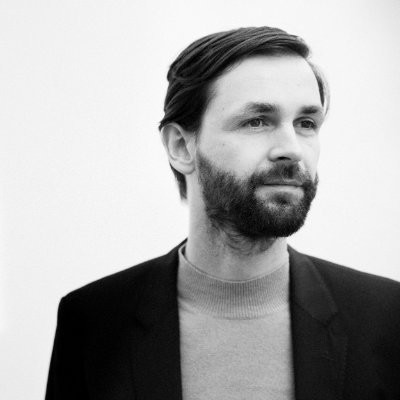
Climate change is one of the most important issues today, if not the most important. According to some, its importance overshadows that of AI, no matter how hyped the technology may be. Some of that is justified. As useful as a smart toothbrush is, as interesting as speculating about superintelligent beings taking over humanity is, those things are pretty trivial compared to the environmental damage caused by greenhouse gas emissions from the products of the industrial revolution: cars, planes, factories. Nonetheless, the claim that AI is less relevant compared to environmental issues misses the close connection between AI and the environment. Smart systems can both prevent environmental damage and augment existing environmental problems.
Take the Green Horizons project, which was started in 2014 by the city of Beijing in cooperation with the company IBM. Using traffic cameras, social media, weather stations, and wearable sensors, among others, data is collected on the distribution of particulate matter, the most dangerous form of air pollution. AI systems are unleashed on this data. Those systems analyze the information received and predict where and when pollution will occur. Such predictions can go ten days into the future, allowing the government to take targeted action to improve air quality. The result? The amount of particulate matter decreased by 20% in a few years, which is a very good consequence, especially considering that thousands of people die every year in China as a result of air pollution.
But AI can also be harmful to the environment. Smart systems are not just intangible entities in the cloud, they have a material impact as well. They require computers to store large amounts of data and make calculations quickly. Let’s unpack what that means.
First of all, producing computers requires tin, silver and other precious raw materials. About 36% of the globally available amount of tin goes into making electronics, and more or less 15% of silver. Mining is extremely harmful to the environment and can have a significant social impact on local populations.
Moreover, the use of those machines requires a lot of energy. An estimated 5 to 9% of all energy consumption worldwide is absorbed by information technology, including AI. Training a large AI system consumes about 2.8 gigawatt-hours of electricity, equivalent to the output of three nuclear power plants in one hour. A related problem is that AI is associated with large greenhouse gas emissions. 2% of global CO₂ emissions are currently said to be generated by information technology and AI. Just training a popular algorithm like those of YouTube emits over 200,000 kilograms of CO₂. By comparison, an average European flight emits about 500 kilograms of carbon dioxide per person into the atmosphere. Researchers Lotfi Belkhir and Ahmed Elmeligi suspect that by 2040, an estimated 14% of global CO₂ emissions will come from smart technology.
Just like any other technology, AI is not neutral, nor is its moral and environmental footprint. So it is truly essential that we include AI in debates concerning climate change. Because the latter goes far beyond the obvious challenges that come with the breeding and consumption of meat, disposable diapers, air travel and driving. We urgently need to broaden that conversation.

.jpg)