What you need to know about AI according to Pieter Abbeel
The first week of April 2017, we took 15 European Insurance experts to San Francisco on an ‘Innovation Tour’ in order to inspire them about their ‘Day After Tomorrow’. This...
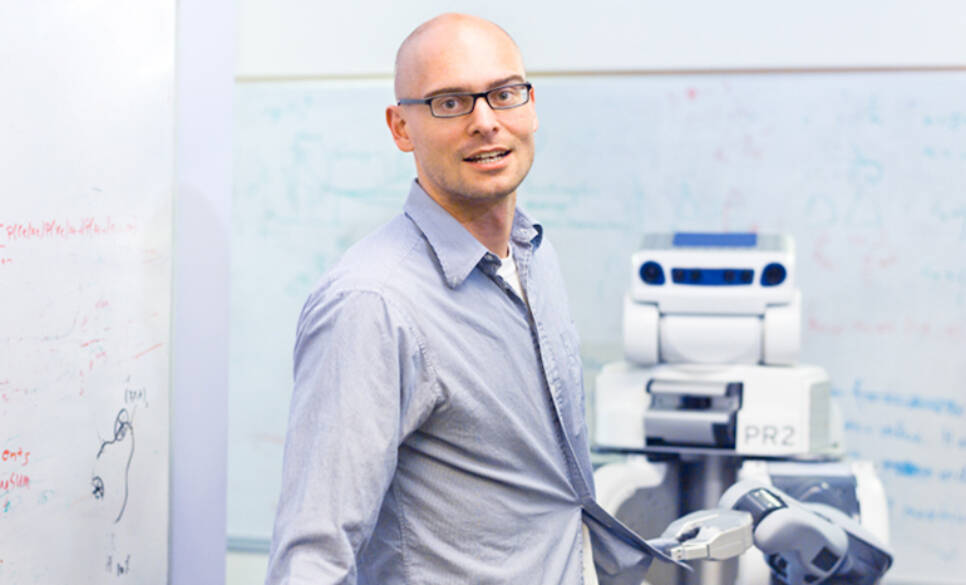
Deep learning research is essential for the proper functioning of robots. Evolution and successes in that area can be summarized in 3 categories, according to Pieter:
- Supervised learning. Basically, you have a lot of input and the system provides an output. It recognizes patterns but you need to give the system labels that it can compare the data with. One well-known application of this, is image recognition. Computers can now tell you how many buses there are in a picture or can describe that there is a girl with a yellow t-shirt in a picture. Downsides? It’s not perfect, it does make errors and it needs enormous amounts of data.
- Reinforcement learning, then, is based on feedback. Once you give a system input, a feedback loop is triggered. The input has a consequence which the system learns to use to update its performance. The task you give the robot, is maximizing rewards. Compare it with a kid doing something, receiving a candy and then learning that this is the correct way. So it starts acting accordingly. Possible applications are dialogues processes, customer interaction services, marketing, etc. Challenges? Stability, first and foremost. If there is no stable feedback loop, your system fails. Secondly, you need a crazy amount of experiences to compare situations with.
- Unsupervised learning is also called the holy grail in AI research. You don’t want to give the kid a candy every time it needs to learn something. Think about a baby, looking at the world and learning from its observations. What does this mean in computer language? You actually send data without a label and the system deals with it AND gives you a correct output. To compare it with the first category of supervision learning which is capable of identifying what’s on a picture, these systems can generate the picture itself. These systems are evolving extremely fast.
All the smart kids are doing it
These AI concepts and revolutions might still sound and look quite abstract. One might be sceptic about the results. It doesn’t look like a Disney World experience. Yet. All the above evolutions are evolutions of the past 5 years. Costs have dropped and the number of extremely smart people deciding to spend their time, figuring out AI, is accelerating.
Elon Musk is undoubtedly one of them. Being one of his Open AI colleagues, Pieter Abbeel asked Elon Musk why he once became interested in AI. Simply he said, well, my cousin is at Berkeley and ALL the smart kids are starting to look into AI. We’re not only talking about computer scientists but about physicians, doctors, mathematicians, chemists, …
The frontiers of the next 2 years
The breakthroughs in AI of the past 5 years are only the icebreaker, making way for bigger ships to thrive in their wake. Here are the likely future disruptions in this area:
- Memory. Now, AI is capable of learning something in different ways but it always starts from scratch. Pieter expects computers to be capable of remembering what they’ve learnt, very soon.
- Shared or transfer learning. Once you’ve taught a robot to put a cap on a bottle, you’d expect it to transfer that knowledge to other, similar processes, such as for instance letting a hammer tap in a nail. This will be another exciting breakthrough to let them learn simulations.
- Speed of learning. Computers can learn things and become extraordinary good at something, even better than humans can do it. However, humans learn it faster today. Pieter’s predicts that via meta-learning, breakthroughs can be expected. Tests during which they train systems to cope with new data extremely fast are reaping success.
- Safety: how do we make sure nothing bad happens with this kind of technology? How do we make sure AI will do what we value as humans? This is a very big challenge we still need to tackle, and it has some of the smartest people on Earth (Stephen Hawking, Elon Musk, Bill Gates, among others) quite puzzled.
- Applications: ask any current VC when the last time was they saw a startup pitch that DID NOT contain AI in their value proposition. Very rare. From drones to self-driving cars, from ordering flight tickets, to planning your agenda. You name it. The question is: are you thinking about AI?
Making sure we’re friends with AI
We’re facing a world in which hundreds and thousands of people will know how to make an AI device. A world in which a company’s stocks fall 2% – losing 1.5 billion dollars in one day – because their head of AI, Andrew Ng, resigns. A world in which data is the only – but solvable – bottleneck.
What will AI look like leaves room for debate. Be it a sort of smart assistant, empowering your brain or a sort of neural dust layer making it smarter– in itself. How exactly doesn’t matter, but we do need to carefully considerate the ‘dark side’ of AI. Because humanity only has one chance at this. It better be in a way that we become friends with it. Because there is no ‘if’ about the world of AI. Only when.
Read the first part with some of the highlights of this tour here.
Want to join us on one of our innovation tours? Check our upcoming tours here.